Programming Note: No blog post next week for Thanksgiving. We’ll be back after that with some content to wrap up the year. To prepare for Thanksgiving, we thought we’d give you a hot take this week, so you have something to discuss over dinner that’s not politics.
2025 is going to be the year that AI has to deliver on all the promise and hype from the last couple years — or else! As such, AI strategies are all the rage today, just like cloud strategies and mobile strategies were before them. Unfortunately, planning your AI strategy is mostly a waste of time, and you should probably stop.
What do we mean? Well, an AI strategy is a way for you to plan how your organization is going to adopt AI — and also of course a way for you to attract investors and appease your board. The problem is that having a single strategy for adopting AI doesn’t make much sense. There are very few principles that apply well across all the possible applications of AI within an organization, so there’s only really one AI strategy that we support: Use more AI. (Yes, we’re biased, but we still think we’re right!)
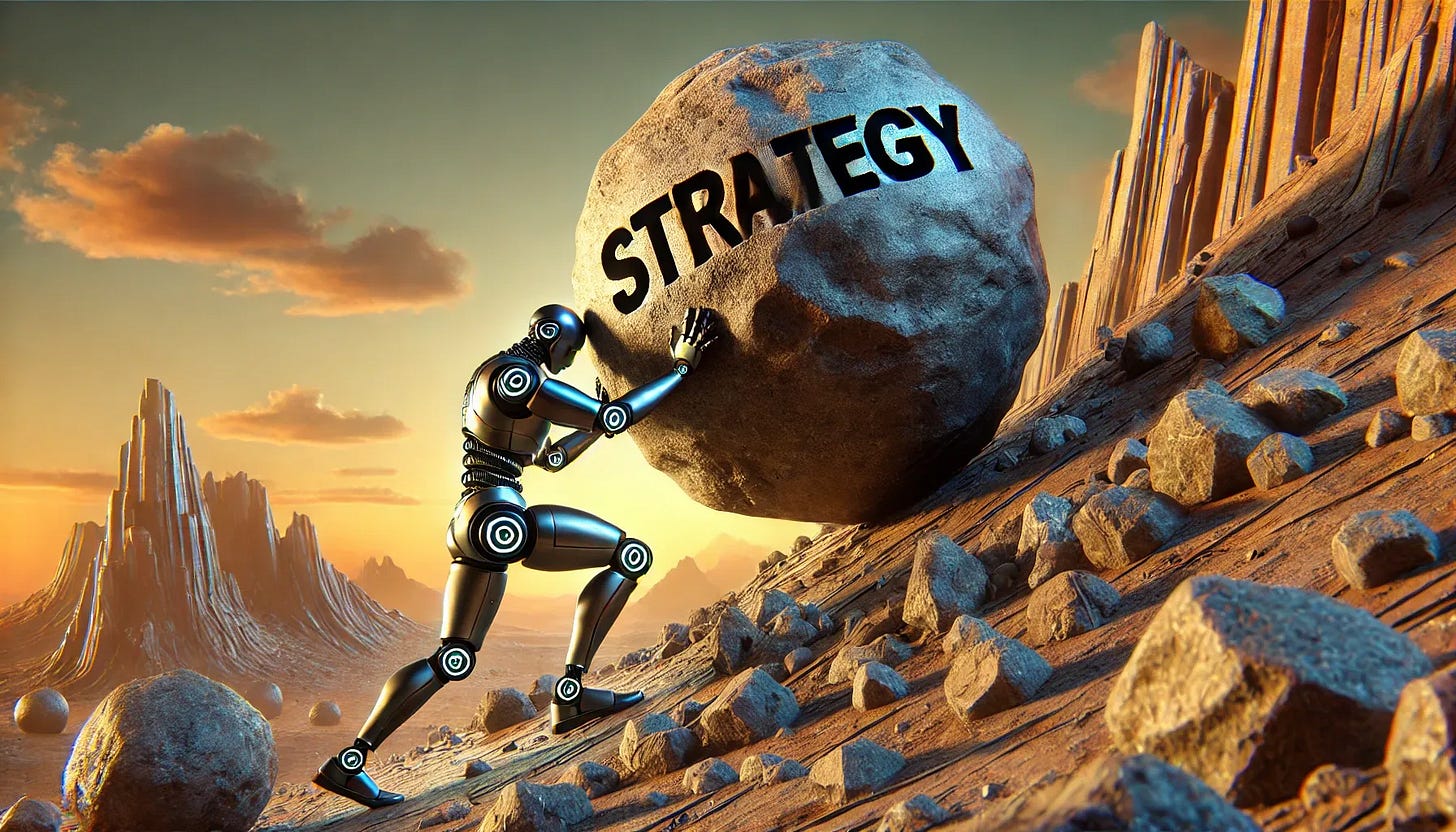
There are many pitfalls that you’ll encounter when trying to develop a single AI strategy for a whole organization, but the one that we’ve repeated in basically every blog post this year is that things are simply changing too fast for anyone to formulate general organizational goals for how AI should be used.
Moreover, we believe (increasingly strongly) that much of the value derived from AI in the near term is going to be from AI application companies. In the same way that you wouldn’t have the same hiring process to evaluate software engineers and account executives, you shouldn’t have similar criteria to evaluate two AI products in different spaces. You also wouldn’t have a centralized committee determine which parts of the business should hire what kinds of people; you would let the head of each department figure out what’s best for them. Dictating that the sales org should adopt AI independent of whether AI sales tools fit their needs is a fool’s errand.
Finally, AI strategies pretty quickly tend to run amok in terms of bureaucratic restrictions. In one of the more frustrating experiences we’ve had, a CISO asked us to fill out a questionnaire about whether we had evaluated the societal impact of our AI tooling. We wanted to yell, “You’re building a database, and we’re answering questions about it!” — but we kept our cool. Safe to say, we didn’t win that customer. This isn’t to say that you shouldn’t be asking this question about some products, but it shouldn’t be a blocker for every use case. This is also definitely an extreme example, but it’s not an isolated one. We’ve had plenty of customers tell us that they have to run all AI purchases by a centralized committee which can take months to review decisions, even for something as simple and common as GitHub Copilot.
With all that complaining out of the way, what should your AI strategy be? As we said above, we think that basically everyone — ourselves included at RunLLM — could be doing a better job of taking advantage of AI. Your strategy should be to use more AI!
More seriously, here’s a few principles that we think are generally applicable. To be clear, this isn’t really a strategy. There’s no centralized process or order of operations here. Instead, it’s a set of guideposts that should help you make clearer and more thoughtful decisions.
Let the experts decide. As we touched on above, centralized committees shouldn’t be deciding which AI tools to adopt. The specific teams — whether it’s sales, marketing, or engineering — who are going to be using the tools should be the ones responsible for deciding what is going to impact their productivity the most. While it might sound obvious, what works in one area won’t necessarily work in another, and the people who are closest to the problem will have the best perspective on what will help the most. This also means avoiding ridiculous questions like the ones above — if an AI tool is writing code or answering technical questions, the societal implications probably aren’t all that vast.
Know how to evaluate. We’ve touched on evaluations many, many times over the last year, and while inconsistent evaluation processes still frustrate us from time to time, we’ve learned to accept that different teams will have different methods for evaluation. What we’ve found consistently fails, however, is teams that show up without any concrete evaluation plans. These folks end up wasting their time and ours, and they almost never end up buying anything. At a minimum, you should know what you’re prioritizing — speed, accuracy, UX, or something else. Even better, you should be able to articulate what you think an acceptable solution is and which categories you’re willing to compromise on. It doesn’t have to be empirical — in fact, it very likely won’t be at this stage — but it does have to be precise.
Encourage using AI! We see far too many of our champions fighting internal organizational battles to get permission to use AI even for internal purposes. We understand some initial skepticism about anything customer facing, but internal tools should be fair game. Of course, there are valid questions around security and compliance that should be answered as necessary, but beyond that, you should be willing to try AI tools out. Putting unnecessary organizational guardrails up is just going to slow your team down and put you behind the competition.
Be willing to accept some failures. Not every use case of AI is going to pan out perfectly. The technology’s still evolving, and some solutions out there simply aren’t very good (yet?). A good salesperson would tell you that if you’re winning every deal, you’re not talking to enough customers. As good AI adoption enthusiasts, we’ll tell you that if every AI purchase you made worked out perfectly, you should probably be trying more. This might sound silly at first, but the technology and market are so early that it’s very difficult to make perfect buying decisions. More importantly, trying out some products that don’t work will make your instincts sharper for future purchases.
We’re very obviously biased, but if you’re reading this, you probably are too. We think more people should be using AI in general, and more people should be using RunLLM in particular. With how quickly things are changing, we think it’s one of those hit-you-over-the-head obvious points to say that the goal should be to get AI into as many areas as it makes sense. If, instead, you’re spending your time on nuanced and complicated AI strategies, you’ll probably find that your plans have become obsolete by the time you’ve finished writing them down.
Wild that the policy mess of algorithmic impact statements have made it to the valley.