We’ve been hearing (and saying) the phrase “the AI market is maturing” a lot recently, but this phrase is really just a thinly veiled way of saying “users and vendors are both figuring out how to use AI.” Until now — in the absence of any real data — we’ve all been relying on analogies to the SaaS products and/or wild guesses to help guide our product decision-making. But as anyone who’s spent even a few minutes with ChatGPT knows, analogies to the pre-AI world only take us so far.
At RunLLM, we’ve been in production for close to a year now and are answering thousands of questions per month, so we wanted to share what we’ve learned about how AI changes user behavior — In many ways, AI is as much of a sea change as was predicted. Many of the intuitions we had about user dynamics we had simply don’t hold in this world.
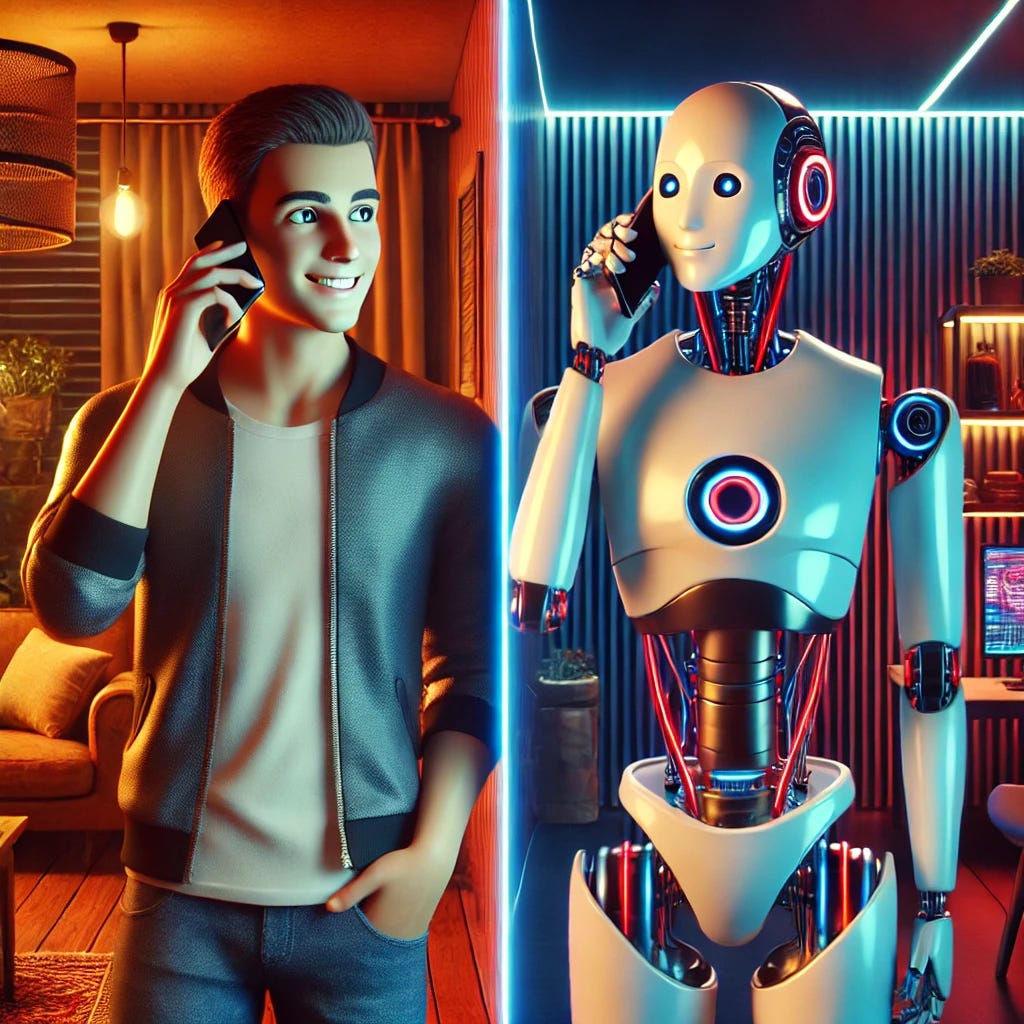
Before jumping in, a few caveats. First, these are our observations from building and selling a single product, so some of the framing that we have here is going to be accordingly skewed — we don’t want to make overly broad claims that we don’t have the data to support yet. Second, the user whose behavior we’re talking about matters a lot — we’re selling to technical audiences that are early adopters, but the same dynamics might not hold elsewhere. Finally, the market is changing incredibly fast, so some of these observations may seem outdated in 6-12 months.
All that said, here’s what we’ve learned.
AI products require (but also foster) a change in mindset. We’ve seen an interesting introductory transition period in every one of our customer deployments. In the same way that SaaS was an obvious but not super accurate analogy for product builders, users tend to approach their interactions with AI products in the same way that they would approach a human support agent. This affects both the content and the volume of the questions that they ask when they’re getting acquainted.
What we’ve seen from the most successful users though is that they quickly transition their mindset into one where they use AI systems to iteratively customize an answer into one that works for exactly what they are looking for. They don’t expect a single prompt to generate the perfect output, and they also know that asking the AI product to iterate with them will be more effective and faster than doing it by hand. This mindset shift allows them to be non-linearly more productive once they adopt the AI tool properly
AI doesn’t replace demand, it augments it. The consequence of the point above is that AI doesn’t just replace demand for existing work but actually creates demand for new work. To give a concrete example from our own experience, an interaction with a technical support engineer might typically go something like this:
User: I’m trying to use your API to do task X.
Support Engineer: To do that, you should use the combination of API 1 and API 2. Here’s a quick example that shows you how that might work: …
In response to this, a user would never say, “Okay, great, now rewrite that code in Python, and customize it so that it has the following configurations…” The support engineer’s job is not to customize the code for you, and even if they were willing to do it, it would probably take the more time than it would take you.
RunLLM instantly changes that dynamic: The cost of customizing the code is tiny, and it’s certainly faster to have an AI do it than it would be to do it by hand. The nature of the product means that it’s going to create more demand than existed before because a user who’s using the product well is going to have it do much more than the support engineer previously would’ve been capable of doing.
AI products have more insights than you realize. Once a user base reorients their mindset and starts using an AI product more, they tend to give it a ton of data that has invaluable insights. As the example above probably illustrates, users are willing to ask AI products to do things that they would never ask a person to do. The same holds true when it comes to asking questions they’d never ask a person — either because they don’t want to waste a person’s time or (more realistically) because they’re afraid that asking a simple question will make them seem silly.
Once an AI tool removes that anxiety, users will ask all manner of questions to an AI, and those questions have invaluable insights: What features is the user using? What is the user confused about? Is there a set of features that users are asking about that aren’t currently supported?
This data simply isn’t available without an AI product because users won’t ask those kinds of questions and a good support representative would be very expensive (and probably get frustrated) if they spent all their time handling mundane, repetitive asks.
From bottom line to top line. The point above is an example of a broader trend in the “maturation of the AI market.” Today, most of the focus on wins derived from AI is on process automation and therefore cost savings. This makes most AI products bottom line-oriented (you increase profits by reducing costs relative to a fixed revenue). This isn’t a bad thing: Theres’s a ton of value to be unlocked here, and we typically explain RunLLM’s value primarily as saving time and money.
However, the next maturity step is in showing customers that AI products can not only save time & money but also grow revenue — in other words, become top line-oriented products. To continue our analogy from above, the user who asks an AI support engineer to customize their code and debug any issues is likely to adopt the product they’re using faster than someone doing those tasks by hand. Whenever any issues arise, they’ll likely be able to resolve their issues quickly — with the AI — rather than sitting with their frustration. Faster adoption and quicker resolution both translate into better customer satisfaction.
That builds trust which unlocks opportunities to encourage users to use new features and get more value out of the product they’re using. This can in turn lead to upsells or simply to better NPS. All of this means revenue expansion in addition to time & cost savings, which is the holy grail for every product to reach.
It’s hopefully not controversial for us to say that the AI market is very early. We have a long way to go, and again, many of the things that we described here might change significantly over the next 6-12 months. If we had to guess, the learning curve will shorten and more users will come to the table with an already-shifted mindset.
We believe the rest of the dynamics we described here will likely hold. The consequence of that is that we should all be thinking about the TAMs startups can access not as static artifacts in Gartner reports but as rapidly evolving markets where the demand is going to grow significantly. Exciting times!
As an ai language model, I have declined your call.